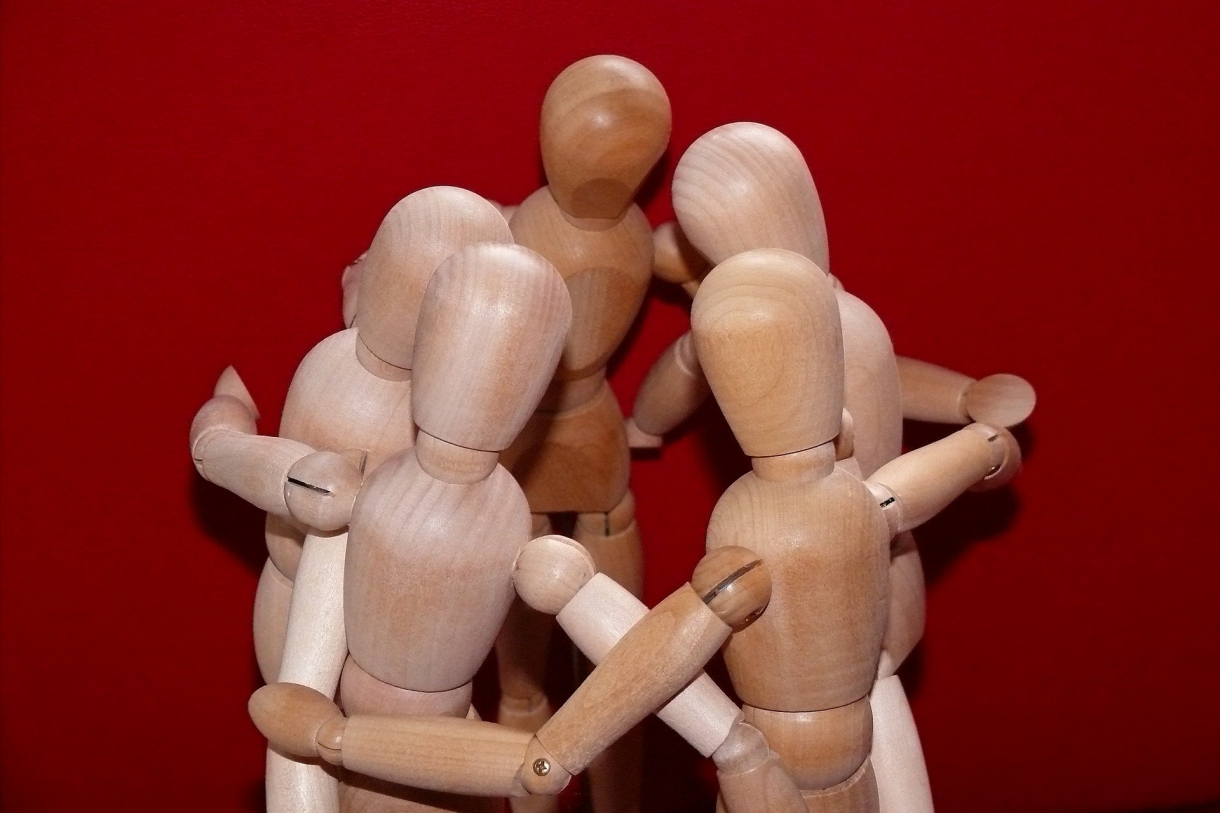
Share this Post
Introduction: The Business Model of Leading Platforms
Online platforms offer their users services that have become an integral part of modern life. From social media to email, search engines and streaming services to online marketplaces, much of our personal and economic activity is carried out online. While some platforms charge their users for services provided (e.g. Netflix, Amazon), others provide their services for free (e.g. Facebook, Google, Waze). Regardless of their income model, nearly all platforms collect huge amounts of data about their users, causing some to state that data is the world’s most valuable resource. This data is stored and analyzed by platforms giving them access to data about their users that can easily be exploited in favor of platforms’ financial interests.
Data collected by the platforms directly from users activity on the platform is not the only source from which platforms gather users’ data. This data is complemented by data gathered from other sources –online as well as offline. An example of how platforms can collect information about their users’ online activity from other websites is the ‘Like’ button embedded by Facebook on various sites, allowing it to track individuals’ activity on a third-party site even if they do not click the button, by adding a snippet of code to the site, and even if they are not members of the social network. Google also tracks its users’ activity on third-party sites, such as news sites, even if those sites were not reached through a Google search. In some cases, this is made possible due to the fact that the third-party site presents ads served by the search engine. Platforms also utilize offline sources, such as data brokers, to learn even more about their users. They can gain access to their users’ buying habits and credit scores as well as location data and voter registration records.
This data is used by platforms in a variety of ways. First, platforms share this data, derivatives of it, or insights gained by its analysis, by providing third parties with access to the data collected (often raising substantial concerns over protection of users’ privacy, or lack thereof). It is not only platforms which offer free services that sell their users’ data; for example, a recent report found that some paid streaming services also shared their users’ data with third parties.
Another way that platforms use data they collect is for training machine-learning models. For example, Gender Classification Systems are a type of algorithm that is fed the data of many users, including their social interactions, surfing patterns, choice of vocabulary, and the frequency of certain words. Based on an analysis of these different types of activity, combined with the gender explicitly provided by these users, the algorithm can be trained to infer the gender of users who have not expressly provided this information, based on their activity.
Finally, based on the data they collect, platforms are able to target personalized content at users based on their activity and personal interests. This personalized content includes suggestions of people to follow, groups to join, events to attend, or movies a user may find interesting. One of the most profitable types of personalized content is advertisements. Whereas in the past advertisers had to cast a broad net and hope to catch people who would be interested in the product or service being advertised based on platforms’ data collection and ability to match between an advertisement and a user, advertising is now much more similar to fishing with a rod and bait, anticipating to catch a particular fish in the time and place it can be expected to show up hungry.
The Relational Nature of Data
While we may believe that each individual’s data is (and should be viewed as) very much their own, and that the right to privacy protects individuals’ control over their own data, reality is very different. In fact, data has a very strong relational aspect. First, many types of online activities involve interactions with other users, making it impossible to categorize a particular piece of data as pertaining to only one individual. When Jane sends Michael an e-mail about Mary, it pertains to (at least) the three of them. Similarly, Albert can tag Henry in a picture posted by George. Moreover, the relational nature of data remains even if one party is not even aware of the existence of the data provided or produced by someone else. For example, genetic data shared by one individual can impact her family members as well. Moreover, the fact that platforms collect huge amounts of data relating to millions (at times billions) of individuals provides them with the ability to infer certain characteristics about individuals even if they have not been provided explicitly. One of the earliest cases of such inferences occurred offline. By analyzing the purchasing patterns of their female shoppers, Target developed an algorithm that was able to identify pregnant shoppers and even to anticipate their due date fairly well. Many people are quite shocked to discover that platforms collect data even about people who are not their users, creating ‘shadow profiles’ for such individuals based on data inferred by the platform or provided by others. In summary, the above analysis demonstrates that under certain circumstances, even people who wish to prevent their data being shared are not able to effectively do so. The relational nature of data creates substantial challenges for individual privacy.
Data Intermediaries
In 2014, Yale Professor Jack Balkin suggested that protection of individual’s data could be supported by imposing fiduciary duties upon certain categories of platforms such as Google, Facebook and Twitter. Balkin made this suggestion based on the special position that such platforms occupy in the data ecosystem, and the power they have over the data of individuals. Fiduciary duties already exist within the context of certain relationships – such as between a doctor and a patient or a lawyer and her client. Members of such professions have special obligations towards the beneficiary (the patient or the client), including a duty of care and a duty of loyalty. These duties require the fiduciary to uphold the trust the beneficiary has placed in them and to act in order to protect and advance the interests of the beneficiary. Fiduciaries must avoid creating potential conflicts of interests between themselves and their beneficiary, choosing the beneficiary’s interests over their own. While Balkin’s suggestion received some support (including several senators who advanced the Data Care Act in 2018, and again in 2021), it has also faced criticism. In particular, David Pozen and Lina Kahn argued that platforms’ interests are so at odds with those of their users that they cannot be expected to meaningfully implement fiduciary duties regarding their users’ data.
Rather than entrusting platforms with inherently self-contradictory fiduciary duties, the challenges arising from platforms’ data collection and analysis capabilities could be addressed by creating a new body within the data ecosystem. This new body would mediate between the platforms and users by representing its members in their dealings with platforms and protecting their interests. It would be in a position to act to reduce the strong imbalance of powers between platforms and individuals. The intermediary would owe fiduciary duties to its members, and not be faced with the type of conflict of interests that Pozen and Kahn pointed out.
The creation of an intermediary is based on acknowledging the relational nature of data and the fact that a collective solution to data protection is imperative. While people individually cannot fully control their data, pooling the data of many individuals together gives them much more power vis-à-vis the platforms. Indeed, the strength of the mediating body would stem from the fact that it represents many users. By pooling together its members’ data and collective bargaining power, this body would be able to advance their interests in a variety of areas pertaining to data, its protection and use.
For example, currently, the privacy policies platforms offer are designed exclusively by the platforms themselves. Generally speaking, platforms’ terms of service are ‘offered’ to users on a take-it-or-leave-it basis, while each individual user has virtually no ability to influence them. A data intermediary would have the ability to negotiate better terms for its members. Terms might include, inter alia, less data-sharing between platforms, stronger privacy protections, increased limitations on what can be done with individuals’ data and even financial remuneration for members’ data.
There remain many issues that require further research, such as questions pertaining to the internal governance mechanism of intermediaries (who should run them, how should decisions be made, will individual members be able to opt out of certain decisions?). Further central unresolved issues include the economic interests that would be at play and the regulatory infrastructure necessary for the successful integration of intermediaries into the data ecosystem.
What is already clear is that new infrastructure that pools together many users has the potential to change the power structures currently shaping the data ecosystem.
The opinions expressed in this text are solely that of the author/s and do not necessarily reflect the views of the Israel Public Policy Institute (IPPI) and/or its partners.
Share this Post
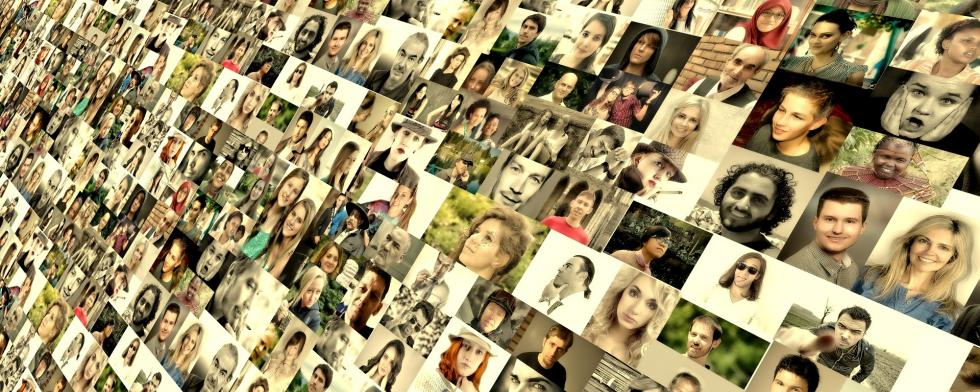
Artificial Intelligence and Democracy
Over the course of the last decade, there has been an enormous interest in artificial intelligence (AI). Due…
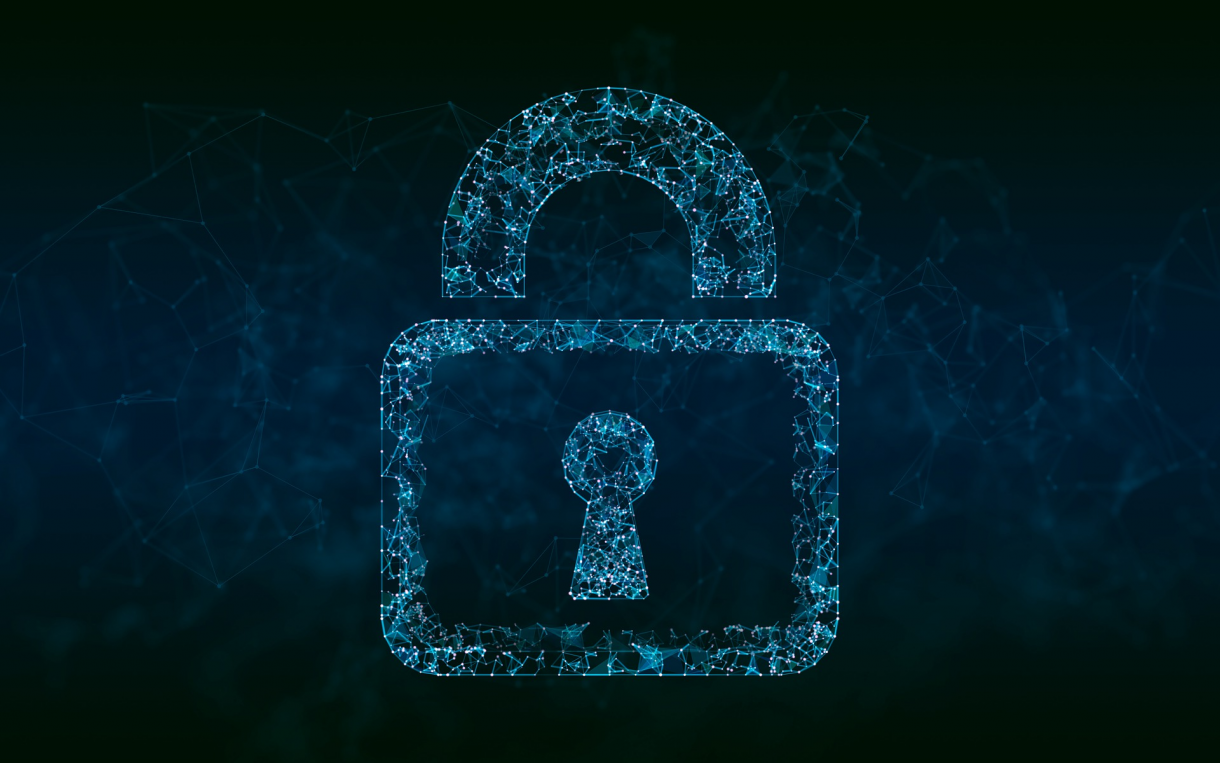
What is Privacy by Design?
The European Convention on Human Rights of the Council of Europe of 1950 acknowledged privacy in its Article…
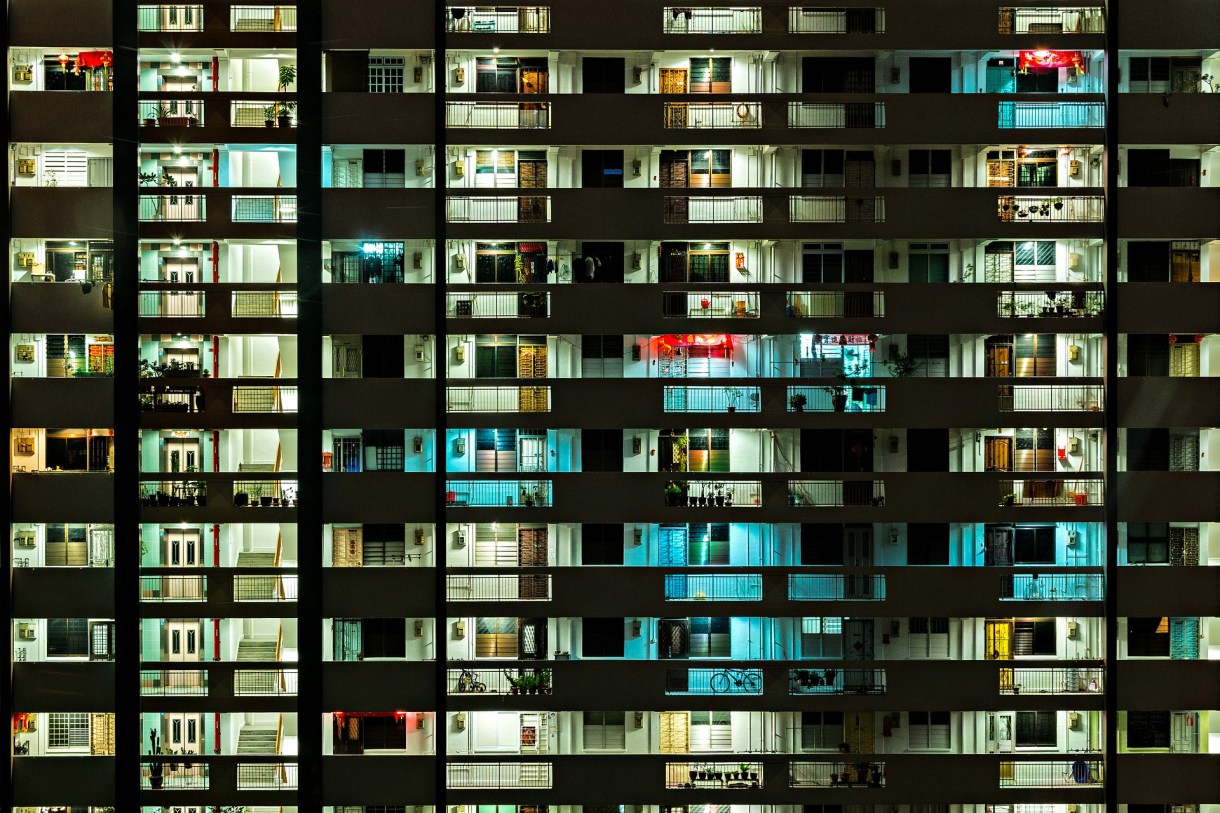
State support for energy efficiency in buildings: A view from Germany
One of the most influential European practice schemes to support energy efficiency investments in the building sector with…