Artificial Intelligence and Tort Law – Who Should be Held Liable when AI Causes Damages?
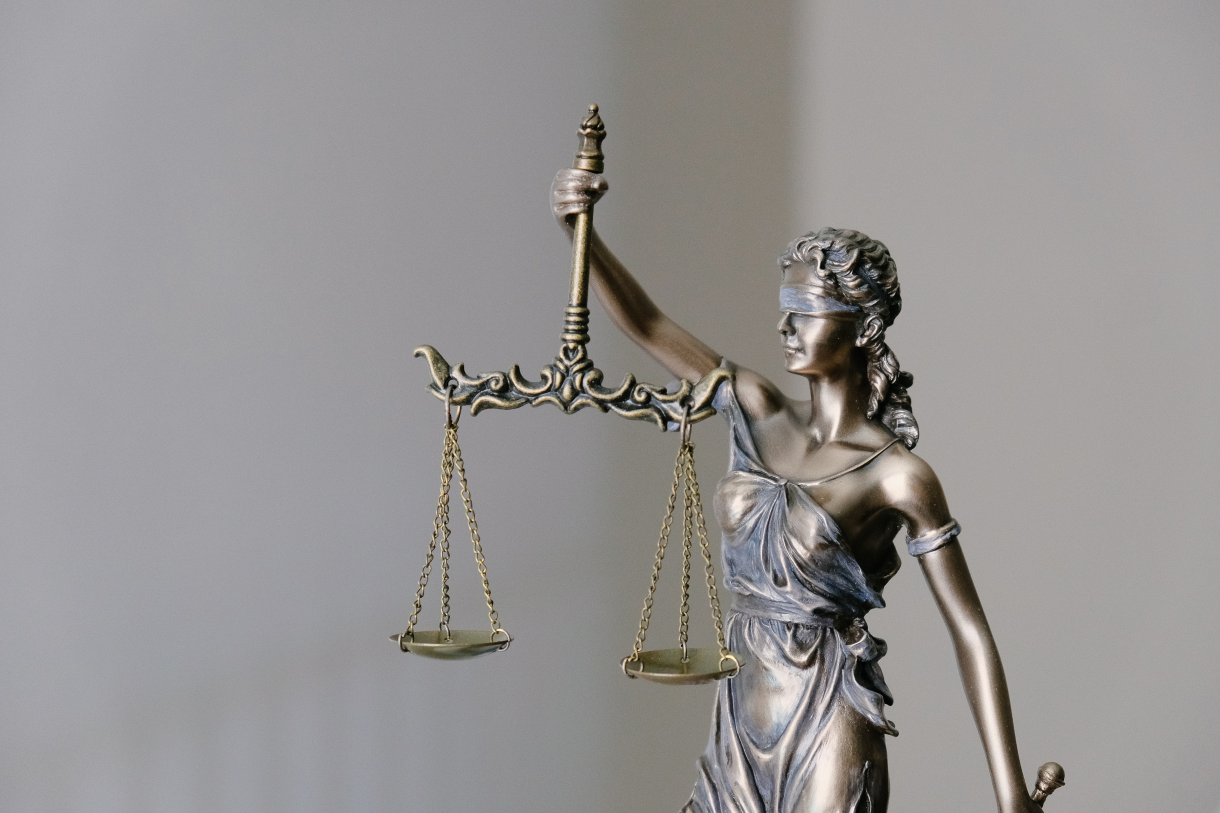
Share this Post
Artificial Intelligence (AI) is becoming a prevalent part of our society and commercial market. Autonomous vehicles, drones, robotic security guards, companion robots and many more are already available for private and public consumers, or are predicted to become available within the next couple of years. However, this emerging technology is not without flaws and raises pressing questions of liability.
A constantly expanding discussion has ensued in the academic world about the appropriate liability regime that should apply to AI entities. While some scholars advocate for a negligence approach, other have called for the application of a strict liability regime, or provision of a ‘safe harbor’ for the creators of AI via ex-ante legislation. This growing discussion reflects the difficulty that emerging technologies, led by AI, have presented to our current tort system and its inability to respond adequately and promptly to technological changes.
However strict the liability regime, it will be necessary to determine just who is liable. Due to the nature of AI, however, this can be elusive, as there is an inherent unpredictability and opaqueness of AI entities’ actions and behavior in carrying out their assigned tasks, given the ‘black-box’ issue.
Regardless of the appropriate liability regime, this spotlight suggests the study of network theory as one possible instrument for enabling stakeholders, such as policy-makers, regulators and the judicial system, to obtain tangible measurements to better identify the human entity that should be held liable once an ‘AI entity’ has caused damages.
Outline
- Introduction
- The Black-Box Issue: An Obstacle to Determining Liability in AI-Induced Damages
- The Current Legal Debate: AI Liability and its Effect on AI Innovation
- A Network Theory Analysis of AI Liability
- Conclusion
Introduction
AI damages come in all shapes and sizes – robotic security guards running over toddlers in shopping centers; AI chatbots making slanderous comments online targeting specific people; and AI algorithms whose hiring decisions are based on discriminatory qualifications. Hovering over these compensable damages, which are actionable in court based on different tort theories, are two main questions – who should be held liable for these damages, and under what liability regime? The answers are not obvious and they occupy the core of an ongoing scholarly dispute. Many individuals operating behind the AI entity can be held liable, whether individually or jointly and severally, for the damages it caused. These include the AI’s owner, programmer, renter, data-trainer, manufacturer, operator, designer etc., and it is not clear how liability should be assigned. Furthermore, it is not clear whether we can view the AI entity as a product and apply a product liability regime, nor is it clear that a strict liability or negligence regime should apply given the unique features, mainly the ‘black-box’ issue, of this emerging technology.
In light of these uncertainties, this spotlight piece suggests using the study of network theory to better equip stakeholders and policy-makers to identify and hold liable the appropriate human entity that stands behind the AI entity. Network theory can also assist policy-makers in deciding the appropriate liability regime once AI liability occurs.
The spotlight continues as follows. Part I will explore the exceptional black-box feature of AI entities. In an AI-liability context, the black-box issue presents a unique problem in establishing causation and naming the liable party when damages occur. Part II will provide a brief discussion of the current legal discussion about the appropriate liability regime and its reciprocal relationship with innovation. Lastly, part III delves into the topic of network theory and provides a methodological framework for identifying the human entity who should be held liable when an AI entity causes damages.
The Black-Box Issue: An Obstacle to Determining Liability in AI-Induced Damages
The black-box issue refers to the fact that the decision-making process of an AI entity can be evaluated neither while the decision is being made, nor in the aftermath of a decision. The latter may be inaccurate in cases where specific actions were taken in advance with regards to the design of the AI entity to ensure evidence can be produced upon demand (relevant in cases where the programmer inserted code whereby the algorithm must log in its decision-making process), as has been suggested by scholars as a partial solution to this problem.
In order to understand the variations of the Black Box issue, it is essential to be familiar with the underlying mechanism of AI and its iterations. AI, explained simply, is a machine, a robot or an algorithm which reaches conclusions and makes decisions without the intervention of humans. There are several sub-branches of AI, all of which involve a ‘black-box’ attribute. Machine learning, a branch of AI, uses the initial code and data base to teach itself the “correct” or “best” decision. As a result, the decision-making processes itself takes place in a virtual ‘black-box’ and is unknown to the human “creator” or user. Neural networks or deep learning, another sub-branch of AI, operates on multiple layers comprised of neurons, and these layers interact with each other through “weighted connections.” The weight of these connections is determined by the AI algorithm and is rarely known or traceable outside of the ‘black-box’ in which the process takes place. The more layers a neural network has, the more difficult it is to fully understand and predict the weight assigned to each neuron and, as a result, the outcome of the AI entity itself. Given these unique features of the ‘black-box,’ neither the users nor the creators can fully understand the process and justification which stand as the basis of an AI decision-making process. (Since the AI entity is self-taught based on multiple complex layers of decision-making, we cannot know for certain who or what is responsible for its final decision.)
When an AI entity inflicts harm or injury, the black box, then, whose decisions are ultimately inexplicable, poses a problem for assigning liability. The lack of foreseeability, the AI entities’ varying degrees of autonomy, and the absence of complete human control with regard to the potential behavior of AI entities lead to difficulty in establishing a legal nexus of causation between the victim and the tortfeasor and a difficulty in reasoning about causation in-fact between the damage inflicted and the liable party. This in turn hampers the attribution of legal responsibility to a specific liable entity.
The Current Legal Debate: AI Liability and its Effect on AI Innovation
The main legal debate regarding AI liability is between those advocating for a strict versus a negligent liability regime. The strict liability camp, of which I am a part, claims that this regime will save the transaction costs of complex and lengthy litigation, and place the financial consequences on the actor in the best position to absorb the costs. It may even encourage innovation we as a society consider to be beneficial, since it is a legal framework that provides manufacturers with certainty and predictability within the field they operate.
On the other hand, the negligent camp suggests creating a new standard of a “reasonable computer.” Proponents of this approach believe that a strict liability regime will stifle innovation. Instead, they suggest a system by which suppliers prove in a cost-benefit-analysis that an AI entity is safer than a reasonable person. Liability will be based on evaluating negligence, which focuses on the AI entity’s actions and not its design. In my view, their suggestion will create a situation whereby once an AI entity is proven to be safer than people, the “reasonable person” standard will cease to exist; rather, our human acts will be held to a “higher” reasonable standard – that of a “reasonable computer.”
This debate is not expected to reach a conclusion any time soon. Regulators and the court systems around the world have not weighed in yet, leaving this discussion mainly in the realm of the academic at the moment.
Regardless of what liability regime is ultimately applied, strict or negligence-based, it will be necessary to determine how to identify the human entity that should be held liable. The remainder of this spotlight is devoted to the question of how network theory can be used as an instrument to identify the appropriate human entity to target for liability.
A Network Theory Analysis of AI Liability
Network theory is the study of symmetric or asymmetric relations between connected entities, or “nodes” within a system, in order to better understand the functions of and interactions between its various components. In the case of AI, a node can be a single algorithm, or even an entire robot, and the relationships and activities connecting these human nodes as well as non-human nodes in the form of AI entities, are called “edges.” These two elements – nodes and edges – present a general view and understanding of a given network, for any specific system a given stakeholder wishes to analyze, as an infinite number of edges (i.e., connections) between nodes. An additional important term is the “boundary,” or the relevant nodes and edges in the network in question. The definition of a network’s boundary is usually subjective in essence, i.e., depending on the perspective of the observer. In a hypothetical AI liability case, the observer might be the judicial or administrative authority analyzing an AI accident, while the nodes and edges in the network might include the victim, the AI entity itself, its manufacturer, programmer etc.
Network theory, in its attempt to describe network relationships, focuses, inter alia, on characterizing the degree of connectivity within a network, and its overall structure (centralized, decentralized or distributed). Connectivity is measured by the minimum number of nodes or edges that can be eliminated in order to isolate the remaining nodes. Much can be learned from evaluating the connectivity level of a given system. For example, the weight of an edge (a parameter meant to evaluate the relative importance of a given edge) and its type (i.e., friendship, trading partners, authority, physical cables, etc.) can indirectly tell us about the strength of the connection, its centrality, and more. We might learn from this, for example, how quickly a new event (e.g., in the form of information) can spread or propagate throughout the system.
The connectivity of a node is directly related to our next characteristic of a system – the centrality of nodes within the network structure. By characterizing a structure (or its topology) by the way relationships between the nodes were formed to determine the relative importance of a node, we can identify the centrality of specific nodes within the network. In other words, if we quantify a node’s “degree” of connectivity determined by the number of edges attached to a single node, we can measure its centrality. The most basic measure of centrality is the standardized degree centrality, which is the number of connections a node has, divided by the total number of possible connections. Another important measure, eigenvector centrality, reflects the connectedness of adjacent nodes to determine a node’s centrality.
In applying network theory to the structure of the Internet, for example, these two measurements would represent the “number of data connections a computer, router, or other device has” (standardized degree centrality), as well as the number of edges these connections have (eigenvector centrality). Nodes with the highest degrees within a network usually have important roles in the functioning of the system as a whole. Connecting the concept of AI liability and the characteristics of a network, the connectivity degree of a node can be a useful indicator for identifying the system’s crucial elements, without which, liability cannot be determined.
Another quantifiable feature is geodesic distance, which measures the minimum number of edges one would have to travel to get from one node to another. This trait provides insights about the nature of the edges’ relationships between nodes and their mutual or nonmutual obligations and commitments. In the context of non-human nodes, these obligations and commitments can be mandated via the code guiding the algorithm to behave in a certain manner.
Structures of networks can be decentralized or distributed. A distributed system refers to a network in which the connections between the nodes were generated randomly, leading to a relatively even distribution of edges. In this system, the relative importance of any node is distributed across the system, and the existence of “hubs” is rarer than in a centralized network. A decentralized network (also known as a small-word network) is generated by local clusters that are connected, but the system is also composed of randomized distant connections. This can affect the position of an AI tortfeasor in a given network and assist stakeholders in analyzing the potential damages an AI entity can inflict upon the system and the human entities connected to it that should be held liable for it.
Another significant feature of a network relates to the weight of the edges, in what is known as a weighted network. In these networks, a specific weight is assigned to the edges among the nodes. This weight indicates the strength of the node and can represent its intensity or capacity. This enables us to further evaluate a node’s strength, in addition to its centrality. In this context, a node’s strength will be the sum of weights assigned to edges that are connected to that node. This is based on the fact that not all edges have the same capacity and that an edge’s weight provides a differentiation measure for its strength, intensity, or capacity. Evaluating the weight of edges between the AI entity and human nodes connected to it enables stakeholders to understand which human nodes have the ability to control and guide the AI entity, making it possible to determine AI liability.
Once an accident involving an AI entity has occurred, we can analyze the features described above in order to better understand the relevant players (i.e., nodes), the nature and strength of the connections between them (i.e., edges), and the importance, significance and role of each in the system and as part of the whole network. The visualization and analysis of a network that contains AI entities is made possible by using these instruments and predefined traits so that we might better control it and regulate it, if we so choose. Considering these network characteristics is imperative to creating efficient and well-thought-out policies.
Once an AI entity causes harm, the network boundaries expand to include nodes that represent the victims, human entities connected to the AI entity etc. The network in which the accident happened (Accident Network) will comprise many other nodes that are linked to it via various types of edges. The two most relevant nodes are the AI entity itself and the victim(s), which are connected by an edge of a “damage” nature. The AI entity will also then expand to include other types of edges, such as ownership, testing, production, programming, training, cooperation, etc. The nature of these specific edges will be considered in analyzing the nature of the relationship as a whole between the AI injurer, the injured parties, and the human entities connected to the AI entity.
Besides the AI tortfeasor (party responsible for injury) and victim nodes, additional relevant nodes integral to the Accident Network and having connections with the AI entity itself may include its trainer, programmer, manufacturer, designer, user, owner etc. These nodes are also likely connected among themselves in various degrees and for various purposes. The tortfeasor and victim nodes will define the boundary of the Accident Network depending on the relevance of their neighboring nodes and the nature of their edges. The Accident Network cannot be infinite. This would undermine its ability to serve as a valuable instrument to identify the liable entity. Nor can it be limited by a predefined, rigid number of edges or nodes. Each Accident Network must be evaluated and delineated in a way that ensures that only relevant nodes and edges are considered.
As mentioned, we are not only interested in the AI node itself, which caused a certain degree of harm, but also in all of the human nodes who stand behind it, are in charge of its operation, and essentially have some degree of control with regard to its tortious actions. The true value of utilizing network theory in the AI liability context is its ability to unmask those neighboring human nodes and their pivotal role in the network, and thus make sure they are held liable for their actions.
When it comes to identifying the entity that should be held liable, it is important to note that AI entities are not independent nodes, but are rather instructed and controlled by human nodes that are connected to the AI node via different types of edges. These human nodes have control and possess the ability to guide and instruct the AI entities. The liable human node(s) in an Accident Network should be identified according to its degree of control over the actions of the AI entity, measured by its capability to monitor, guide, and give directions to the AI node according to the nature and weight of its connection.
AI and human nodes are integrated into the Accident Network and are critical for our ability to identify the party who should be held liable. Inherently, AI nodes possess a high degree of connectivity, and as such they are considered central and are attributed with a ‘high weight’ in an Accident Network. Their actions may negatively impact many human nodes that are connected to them directly or indirectly.
To conclude, the connection or connections between a human node and an AI node in a given Accident Network is best visualized, explained, and analyzed via the use of the Accident Network itself. Analyzing the features of a tortfeasor AI node and the human nodes connected to it provides an invaluable tool for drawing the necessary legal nexuses between the damages, and the tortious acts that lead to them. This is essential, whether a strict liability or a negligence regime is applied.
Conclusion
This spotlight piece suggests that we link AI liability and network theory. Utilizing the analytic insights of this theory, one can apply them when damages and injuries are caused by AI entities, in order to hold the appropriate human node liable.
The broader question of which liability regime should be applied in the AI liability context is a difficult one to resolve. It will take time until legislatures and the judicial branch, in Israel and around the world, will provide insights or guidance. Until then, network theory can assist in identifying the human entity behind the AI tortfeasor node in a manner that enables decision-makers to hold the appropriate entity liable for its actions.
This Spotlight is published as part of the German-Israeli Tech Policy Dialog Platform, a collaboration between the Israel Public Policy Institute (IPPI) and the Heinrich Böll Foundation.
The opinions expressed in this text are solely that of the author/s and do not necessarily reflect the views of the Israel Public Policy Institute (IPPI) and/or the Heinrich Böll Foundation.
Share this Post
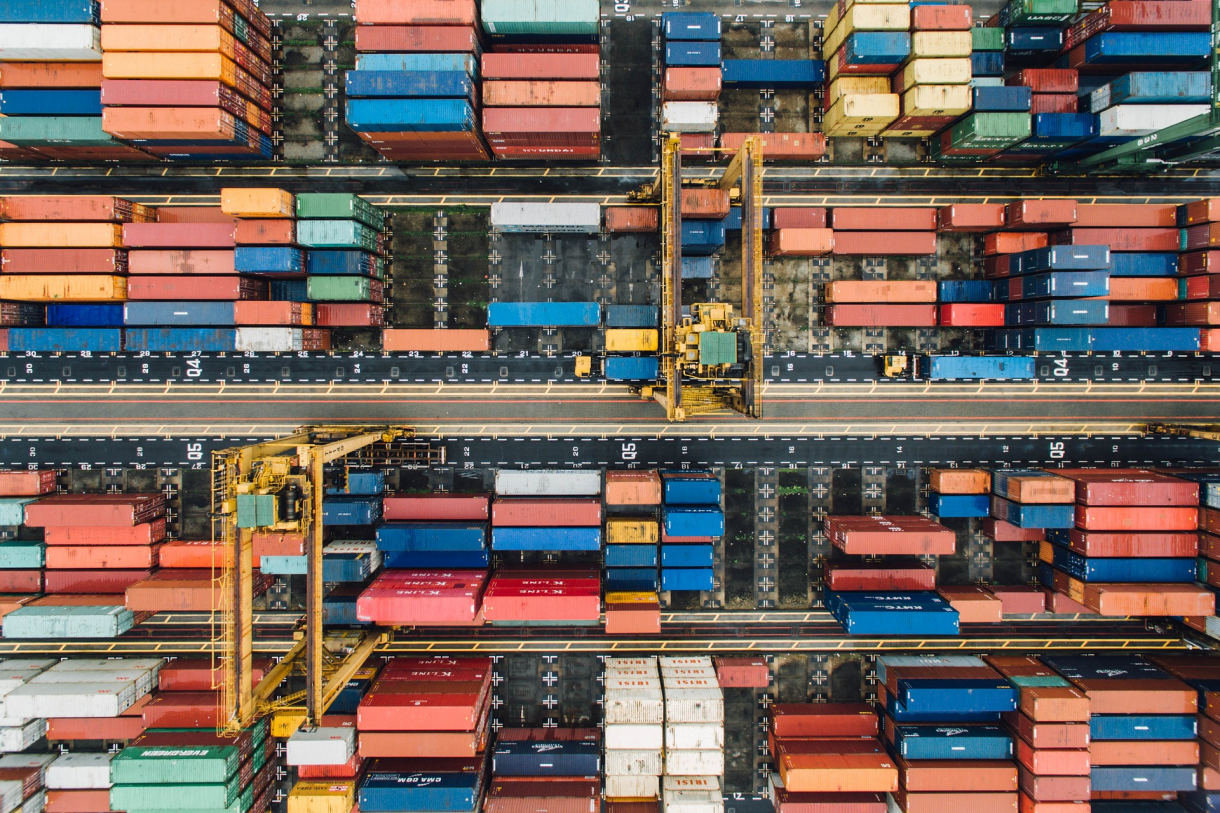
What is the EU’s Carbon Border Adjustment Mechanism (CBAM)?
Varying levels of ambition in different regions of the world can become a serious obstacle to upholding a…
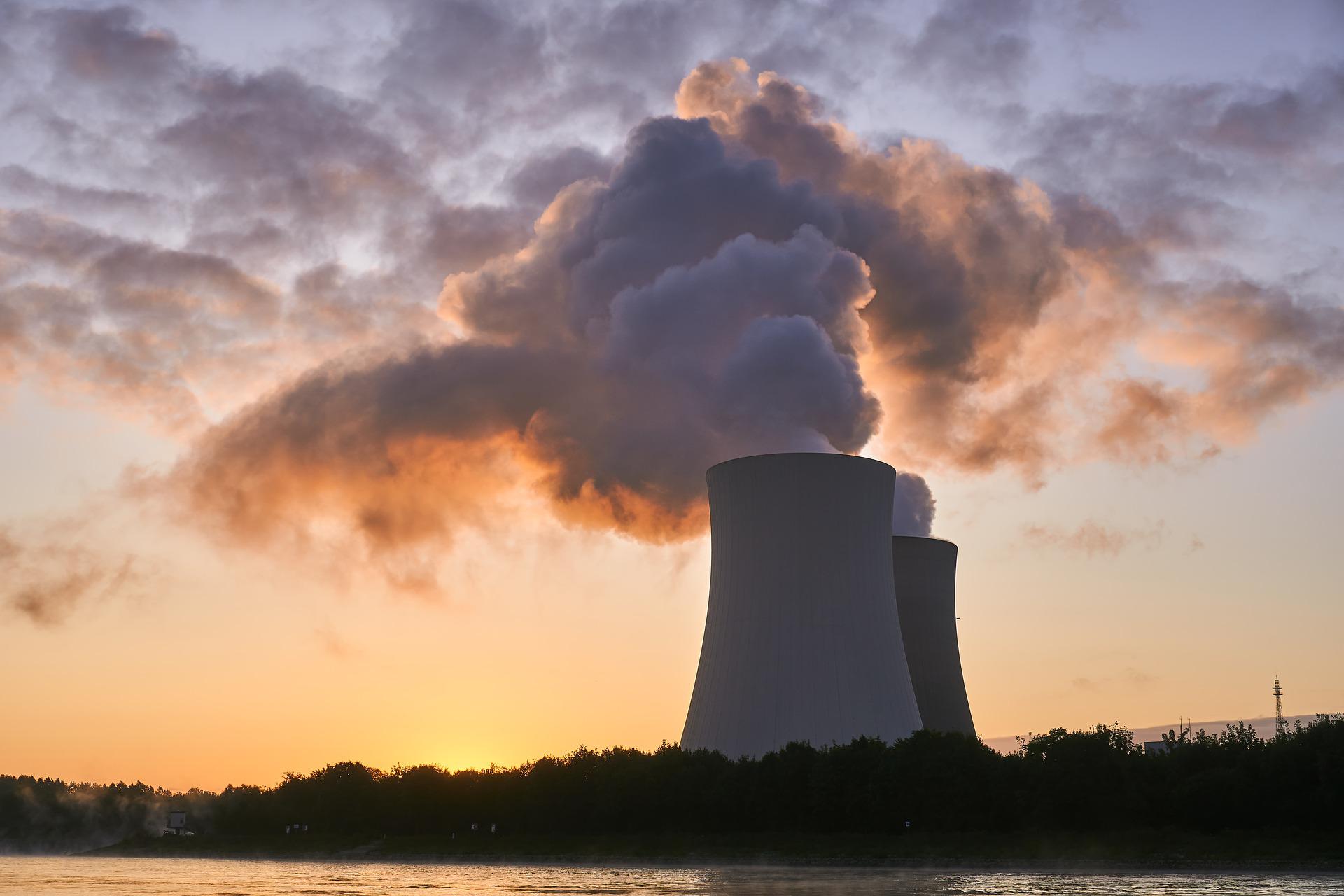
The Nuclear Debate and Energy Taxonomy in the European Union
The new EU energy Taxonomy Regulation, presented at the beginning of 2022, includes certain nuclear activities. Since the…
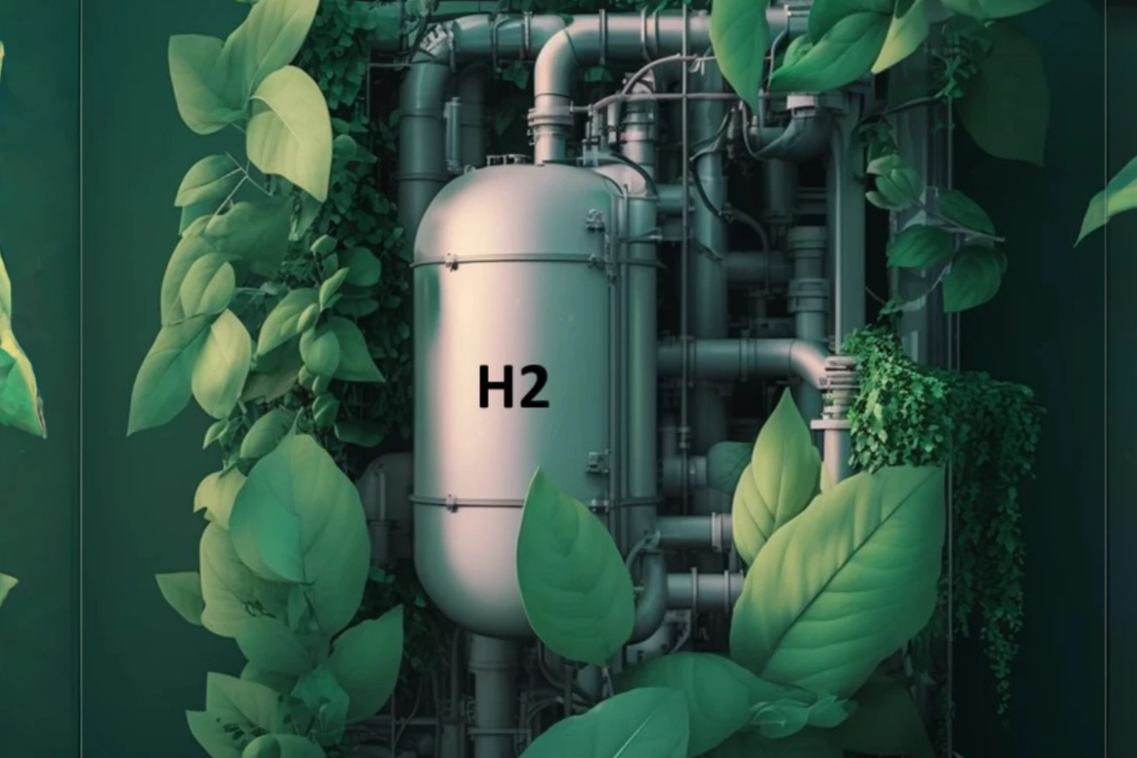
The Promise of Green Hydrogen: The Future of the Global Energy Transition?
Green hydrogen is a zero-emission alternative to fossil fuels, produced by using renewable electricity to split hydrogen from…