Algorithmic Bias in the Public Sector – A View from Austria
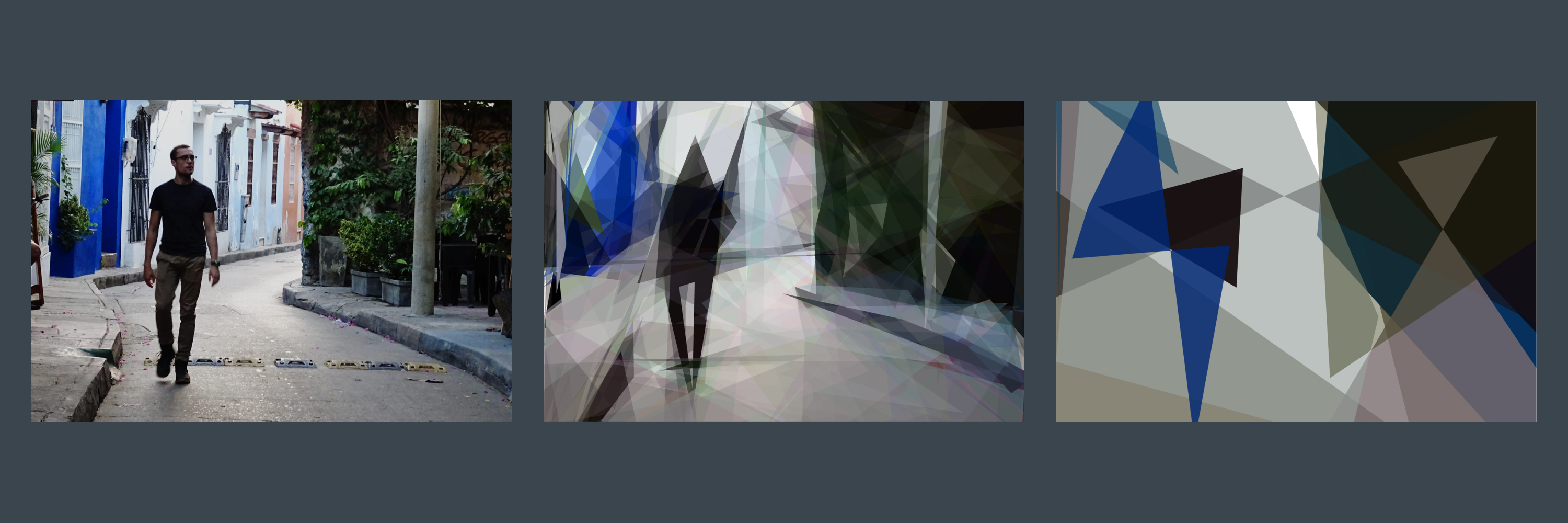
Share this Post
At first glance, an algorithm may be perceived as a more objective judge than a human. An algorithmic decision can be based on big data, through which the algorithm uses learned patterns to predict behavior. Based on this information, the algorithm makes inferences about the user in the future and evaluates potential human performance. The downside of this presumed algorithmic efficiency is that these inferences may include decisions based on racial, ethnic or gender stereotypes because the algorithm might have learned discriminatory behavior from the data used during the system creation, learning or validation phase. The application of such a biased system is especially problematic because it discriminates systematically on a large scale. Systems that have embedded bias might be used for such purposes as predictive policing, advertising that amplifies online gender discrimination, and the distribution of racist information.
The use of biased algorithms can be retroactively prosecuted by anti-discrimination law, or be restricted by privacy regulations such as the General Data Protection Regulation in the European Union. One way of redressing this problem is the growing practice of decoding algorithms. Decoding algorithms is described by Lomborg and Kapsch as “to probe how people come to know and understand algorithms, what they imagine algorithms do, and their valorization of and responses to algorithmic work in daily media use.” However, there are also hybrid system designs in which algorithms are used in a semi-automated process that can be referred to as decision support systems. Such decision support systems should help a human to make a decision and are designed as a stage in the overall process. In some systems of this type, the decision support systems do not make the final decisions, but rather, act as an ex-ante tool for humans before the human in charge concludes the final decision.
A special area of application of decision support systems of this type is the field of Human Resources (HR) in the private, public, and semi-public sector. The use of such a system in this context is often viewed as a solution to save resources. Austria’s Public Employment Service (AMS), which is constituted by national law, created such a system in 2018 to classify applicants and calculate individual scores to determine how to allocate different forms of support (e.g. funding for educational measures) to them. To this end, with the help of a private company, the AMS developed a linear regression model that automatically classifies job seekers and assigns them to one of three possible prospective employee groups.
The Data
The data that determines the AMS algorithm by selecting the attributes and variables on which the model is based uses historic labor market data. This data is not made public and cannot be rigorously assessed by researchers, NGOs, or other institutions. However, a study by Alhutter et al. managed to reveal various other data sources the algorithm uses. Firstly, as part of the registration process, the AMS asks applicants to provide personal data via an online tool. Secondly, the data collected about the AMS customer is added to information collected about the person from the Main Association of Austrian Social Security Institutions.
There are a few limitations inherent in this data. First, the system includes only data about the unemployed, but not about employment opportunities, such that it may not present a holistic picture of the employment market.
Second, the data the AMS collected about a job seeker might be incomplete. This could occur, for example, due to a “fragmented” vita, or gaps resulting from long periods during which data could not be collected, which could lead as well to skewed outcomes and possible negative consequences for the AMS’s customers that no one can alter or influence, including the job seekers themselves. Furthermore, gaps occur in the case of job seekers who were previously employed in other countries. Here, an accurate representation of the job seeker’s chances can be problematic since the data is available abroad but does not appear in the algorithm’s data pool. Alhutter et al. further point out that data is lacking for the demographic group of young adults. Job seekers applying through AMS who are inaccurately represented are more likely to be classified in the system such that they qualify for the least resources. The available employment? data in use at the time the study was carried out, moreover, was collected before the COVID crisis and therefore does not illustrate the current situation accurately. It would, for example, predict high chances of finding a job as a cook, while many restaurants might have closed because of the COVID-19 crisis or stopped hiring because of economic uncertainty.
The Model
The AMS classification system determines whether the AMS should approve an investment in a given job seeker in the form of courses or other educational opportunities, by assigning the applicant to one of three groups. The first category (Group A) grants the customer high chances of re-employment, the second grants medium chances in the labor market (Group B) and the third grants low chances for the unemployed person to find a new position (Group C). The influence of the algorithm is somewhat mitigated by the provision that a (human) caseworker can petition and override the system’s decision.
The tool that determines group placement is constructed, as mentioned, as a linear regression model. Multiple variants are used to calculate a score for the AMS applicant, predicting his or her prospects to find reemployment in the time frame beginning 90 days from the day of application to the following next seven months (short-term) or for the more distant future, to be reemployed beginning 180 days from the day of application ranging to the next two years (long-term). Customers who are assigned high chances of finding a new position in the short term are assigned to Group A, while Group C illustrates the long-term scenario. Group B, on the other hand, is the class that represents the remaining job seekers that do not qualify for group A or C.
Therefore, Group A includes cases in which a job seeker is assigned a high value of more than 66% in a short-term scenario, Group C comprises job seekers with a low score in the long-term scenario with a probability under 25% and Group B is the collection group for the remainders.
The value ascribed to the job seeker is referred to as the IC score, which influences classification via the variables the system uses. Those variables include, inter alia, sex, age, group of states (Austrian, EU member state, non-EU member state), education, health impairment, care obligation and previous career. These variables may not accurately convey the status of the job seeker. For example, for the value “education” there are only three subcategories that the job seeker can indicate: whether a mandatory school level was completed, if an apprenticeship or vocational middle school was attended, or if any other higher school or university was attended. This definition of education does not give the job seeker the possibility to indicate, for example, the details of higher education, i.e. whether only a bachelor’s degree, a master’s or a PhD was completed.
Alhutter et al. demonstrate that the rigid borders between the groups A, B and C that lead to the risk of inaccurate classification.
The system’s design feature whereby human input is included to legitimate the algorithm’s outcome – often referred to as the human-in-the-loop problem –raises an additional difficulty,. Although one might think that the human decision-maker’s interaction with the system would have an ostensible benefit, the person included in the decision is actually not likely to disagree with the recommendation at hand . In the present case, for example, the human, a caseworker at the Austrian AMS, would be more likely to agree with a customer’s classification than to disagree with the system. This systematic lower rating of certain groups places the fair allocation of governmental investment at risk, potentially leading to an increase in inequality in educational interventions for women and other disadvantaged groups in the labor market and reinforcing old stereotypes. However, the system’s transparency – its variables, process and recall data that determine educational interventions for job seekers – is too limited to enable a full analysis of the system.
This design feature, incidentally, might prevent Art 22 of the General Data Protection Regulation (GDPR) from applying, since it means that the process not fully automated; this question remains a matter of scholarly dispute.
Potential Discrimination?
The model algorithm is prone to negative ratings for legally protected categories like gender, race, ethnic origin or age, due to certain built-in features. For example, female users have their score reduced just because of being female in the first place and if they have an obligation of care, a deduction of points a male user does not have to face even if he has an obligation of care. This aspect of the system design is problematic, as the deduction of points for female customers might lead to a broad structural unequal treatment between the sexes. Furthermore, the variable “sex” is designed only in a binary way, leaving no third category of gender as an option for the users.
Deductions because of other attributes e.g. age or nationality can also be found in the system documentation. If these indeed result in negative ratings for protected characteristics, the system could conflict with four European anti-discrimination directives: The Racial Equality Directive, the Directive on Equal Opportunities and Equal Treatment of Men and Women, the Gender Access Directive and the Employment Directive. However, since these European legislative tools have to be incorporated by the member states into national law, they are not necessarily directly applicable.
In particular, Article 3 of the Racial Equality Directive, which prohibits discrimination due to race or ethnicity in the recruitment process, the workplace, the welfare system, social services or cases addressing education could apply to the AMS algorithm’s use. Within the discipline of anti-discrimination law, two categories of discrimination can be distinguished: Direct and indirect discrimination. The first refers to cases that include a protected characteristic, including the mere perception that a protected characteristic is included, or the existence of a connection is made between the discriminated and the person that has a protected characteristic. This might occur, for example, as a result of using the algorithm because of the inclusion of gender as an attribute with a negative score.
The second form of discrimination addresses cases that concentrate on policies or provisions that disadvantage people disproportionately compared to another group, including proof of the disadvantaged treatment in the future or past (prima facie); in these cases, the organization applying the rule is given the opportunity to justify its behavior. An example might be the use case of the variable “sex,” which has been given a negative score only for females, but not for male employment seekers.
The algorithm might seem objective in including this variable, but through the deduction of points only for women, females might be disproportionately disadvantaged compared to the other category of employment seekers – men.
Both forms of discrimination – direct and indirect, in turn, can lead to the promotion of unemployed men compared to women through the use of the system. The discrimination might manifest itself also in the reduced chances for women compared to men for the allocation of educational resources. The resulting skewed allocation of resources could also manifest later in the job market after the algorithm is finally implemented, qualifying men even better in future predictions by the system.Usage of the algorithm could therefore reinforce existing inequalities e.g. for women or elderly people, and even reproduce stereotypes such as counting care obligations only for women as a negative value, thus reproducing and reinforcing the stereotypical image of the housewife as the default parent responsible for the children.
The use of the algorithm furthermore might be in contravention of the Austrian constitution’s stipulation that men and women should be treated as equal rather than unequal. By reducing points for only one sex as explained in the examples above, the algorithm by not treat all humans as equal, and could also lead to a contravention of Art 20 or 21 of the EU Charter of Fundamental Rights. Furthermore, the problem of the binary category for gender might be not compliant with the law. For three years Austria has legitimized a third gender that might conflict with the fact that the system provides only two options in its design.
The Call for Accountability and Transparency
Transparency is a recurring demand as far as the usage of algorithms is concerned.
This demand includes complete information that is needed to truly assess the AMS algorithm and to decide whether taxpayers’ money is being well spent on such a tool. Furthermore, transparency standards require that decisions inferred about the job seekers have to be understandable and explainable to the general public and policy makers, ideally before they are used in the real world without proper consent of the people being scored. Ex ante testing could have the form of algorithmic impact assessments. Algorithmic impact assessments are used to test an algorithm before the system is implemented in real-world scenarios, in order to reduce harm and to gain a better understanding of the sociological impacts that the algorithm or other socio-technical system might have. For example, the Canadian government has built a tool for algorithmic impact assessments that is designed to curb the risk an automated decision (support) system might pose to individuals; conducting such an assessment is obligatory in Canada in cases of administrative decisions about a person. In the case of the Austrian employment bureau, documentation of the system is insufficient to allow for an in-depth algorithmic impact assessment..
A measure to indicate the effectiveness of an algorithmic system for testing is precision. The precision of an algorithm, carried out based on documentation of the system, represents how many of the “predictions” in the respective group turned out to be correct in retrospect. The AMS algorithm would be considered precise to the extent that it can accurately determine the group A, B or C to which a job seeker is assigned. The reported precision of the AMS algorithm, as it turns out, varies from group to group; for example, the precision of the classification to Group C is indicated in the documentation of the developing company is relatively high, between 81-91%. However, even if the precision of the system for group C might be high, there is still a margin of error, which can result in false classification whereby a member can be incorrectly assigned to Group C, leading to a reduced chance of being allocated training, education, and resources. False positives in the context of classification result in job seekers being e.g. assigned to Group C that should have been classified as Group A or B, while false negatives refers to applicants who should have been an instance of Group C but are qualified as either B or A. The scenario of the wrong classification to Group C – a false positive –might be more harmful than the occurrence of false negatives from a user perspective. Given the precision score, such misclassification might occur for thousands of job seekers. And this cannot be redressed, as a job seeker has very little control over his or her classification for many of the attributes used in the system.
An additional critique has been levelled regarding usage of the customer’s home address instead of the AMS location preferred and chosen by the job seeker, which could lead to a reduced IC score.
Generally, it is also problematic that during the process of system creation, key stakeholders including like unions, NGOs, researchers, customers, job seekers and employers have not been included in the development, implementation or testing of the algorithm.
The Algorithm’s Judicial History
After critique of the AMS algorithm was made public, the Austrian Data Protection Authority began questioning the validity of the system. It stopped the usage of the algorithm in 2020. The Austrian Data Protection Authority argued that a legal basis according to §§ 25 (1), (29) and 31 (5) AMSG must be created for the AMS to provide authorization for data usage, that might contradict Art 4 GDPR and Art 22 GDPR accordingly, before re-application of the algorithm.
The decision by the authority was subsequently challenged by the Federal Administrative Court, allowing the system to be reinstated.
Now, however, the Administrative Court of Austria (the highest instance) must decide if the use of the system is within legal boundaries or if the algorithm violated the law and should not be used at all in its current state.
In addition to being subject to critique by the Austrian DPA, the AMS algorithms may further constitute a high-risk application according to the European Commission’s draft for the regulation of AI. The new legislation would introduce, according to the current version of the proposal, obligatory “adequate risk assessment and mitigation systems; high quality of the datasets feeding the system to minimize risks and discriminatory outcomes; logging of activity to ensure traceability of results; detailed documentation providing all information necessary on the system and its purpose for authorities to assess its compliance; clear and adequate information to the user; appropriate human oversight measures to minimize risk; high level of robustness, security and accuracy.”
Whether the AMS algorithm can be classified as an Artificial Intelligence (AI) system that would open the scope of upcoming European regulation remains unclear. While researchers like Adensamer argue for categorizing it as AI under the EU AI Act, the AMS itself claims that because it does not include techniques such as machine learning or neural networks in its design, it should not come under the jurisdiction of the future regulation.Imagining an Optimistic Future
To summarize the problem of biased algorithmic processes: Not only humans might have biases; algorithms can be biased too. Therefore, the decision as to whether a process should be automated or semi-automated is a crucial question for society, and should involve many stakeholders in order to better understand needs and to minimize harm. When a decision is made to implement automation, the design of a system must conform with the law. Compliance with anti-discrimination law is of great importance, especially within a setting such as resource allocation in the job market, if taxpayer money is used for the development, or if administrative resources are dependent upon an algorithmic categorization.
As mentioned, the demand for greater algorithmic accountability and transparency measures is on the rise. To meaningfully implement these demands, organizations have to open up their systems’ source code, data and documentation to researchers, NGOs, journalists and to a certain extent directly to the public at large. Public engagement is a crucial tool for bringing about changes in laws and regulations. Specifically, in the case of the Austrian AMS algorithm, a group of organizations, stakeholders, human rights activists, and researchers launched a petition. This coordinated public action, as well as the previous decisions by the authorities and courts, can be interpreted as a slight change in awareness and the discourse towards higher standards of accountability of algorithmic system use and the potential ban of application areas, or design decisions, as well as a harbinger of future legal mechanisms addressing algorithmic systems at large.
The opinions expressed in this text are solely that of the author/s and do not necessarily reflect the views of IPPI and/or its partners.
Share this Post
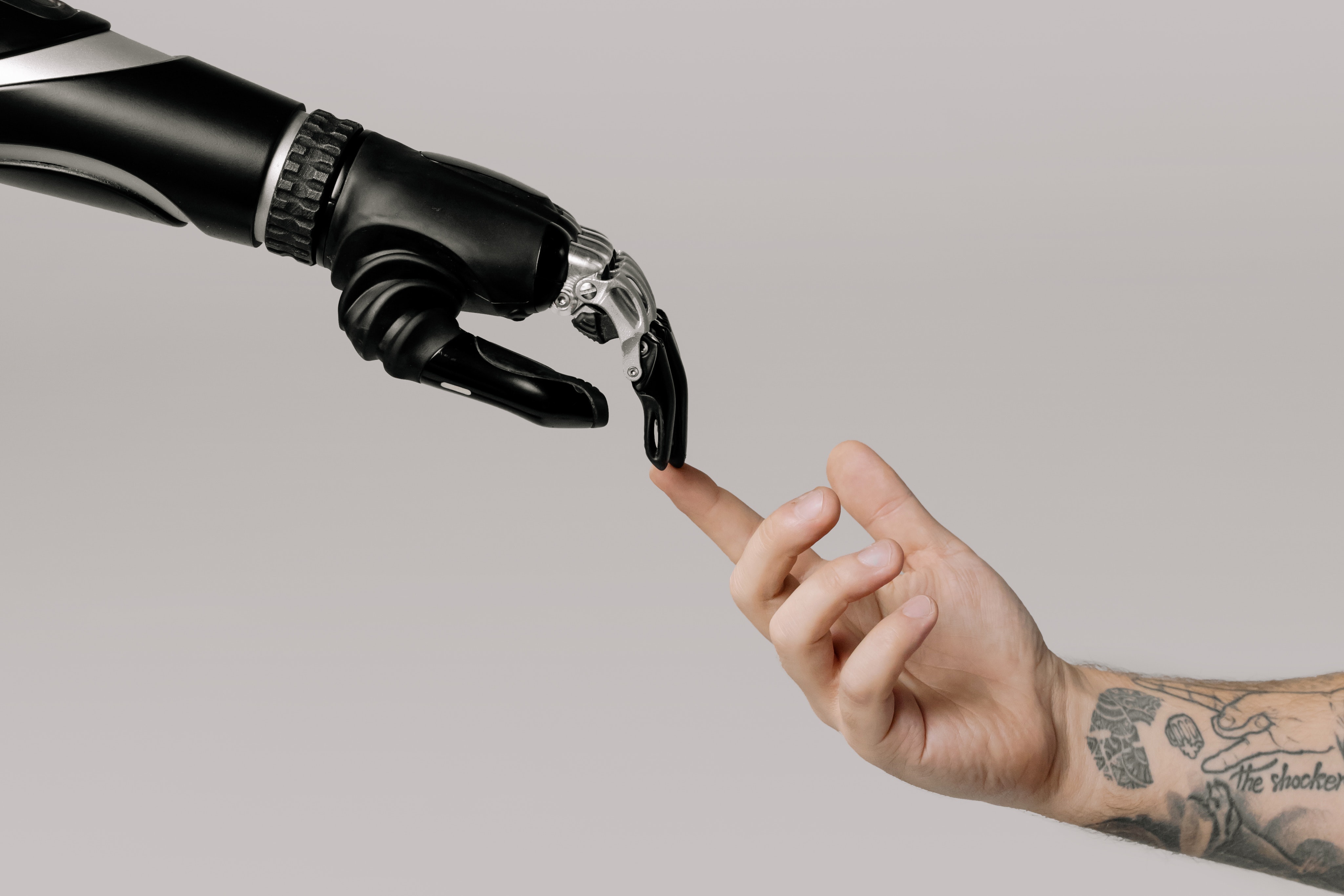
Why 'Responsible AI' Is Good for Business
Artificial Intelligence (AI) algorithms have gained a growing influence over our society. It is not unusual to see…
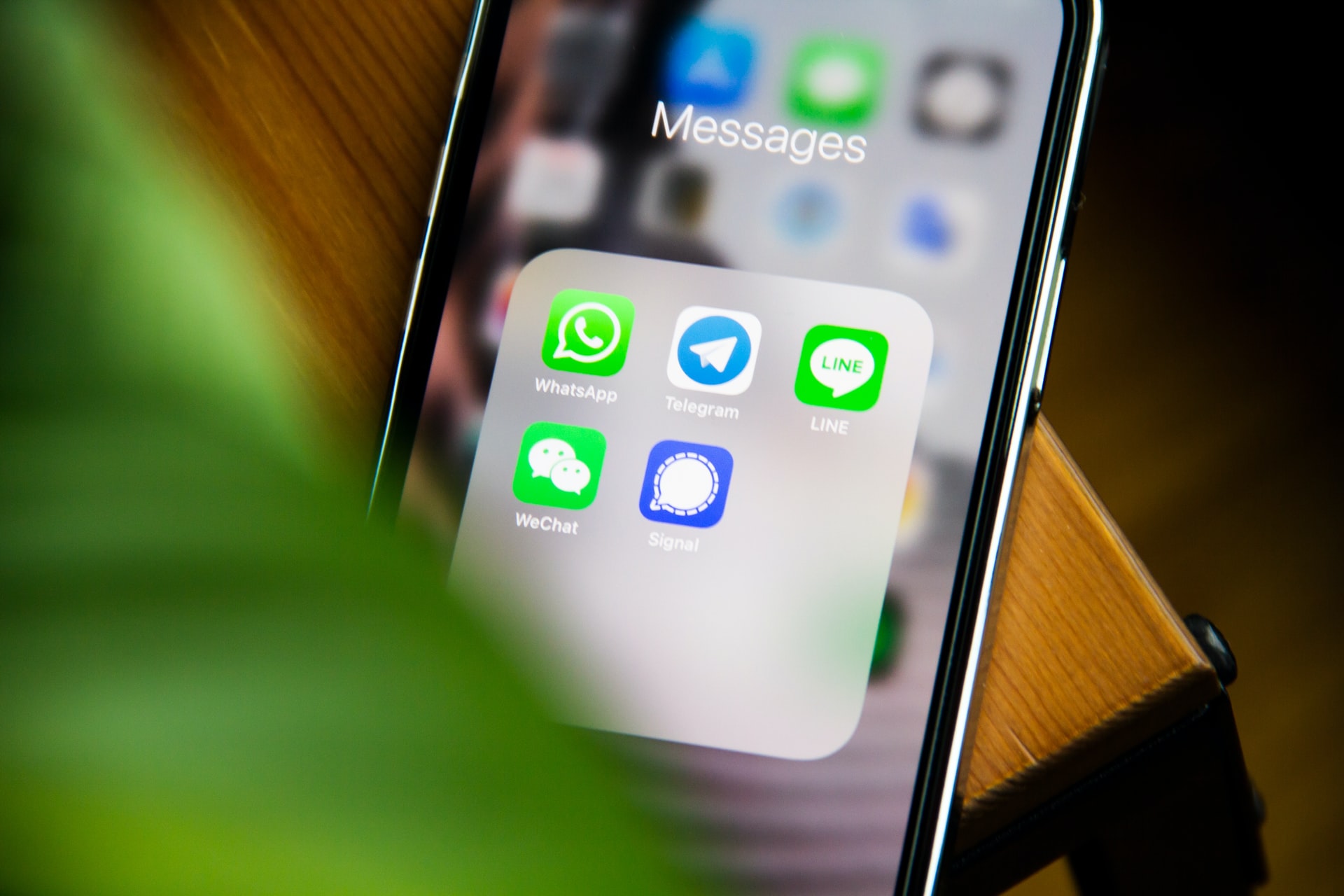
Privacy by Design and Competitive Advantage: a Whatsapp Exodus?
Authors: Eduardo Magrani and Lucas van Hattem The start of the new year might not have taken the turn WhatsApp was hoping…
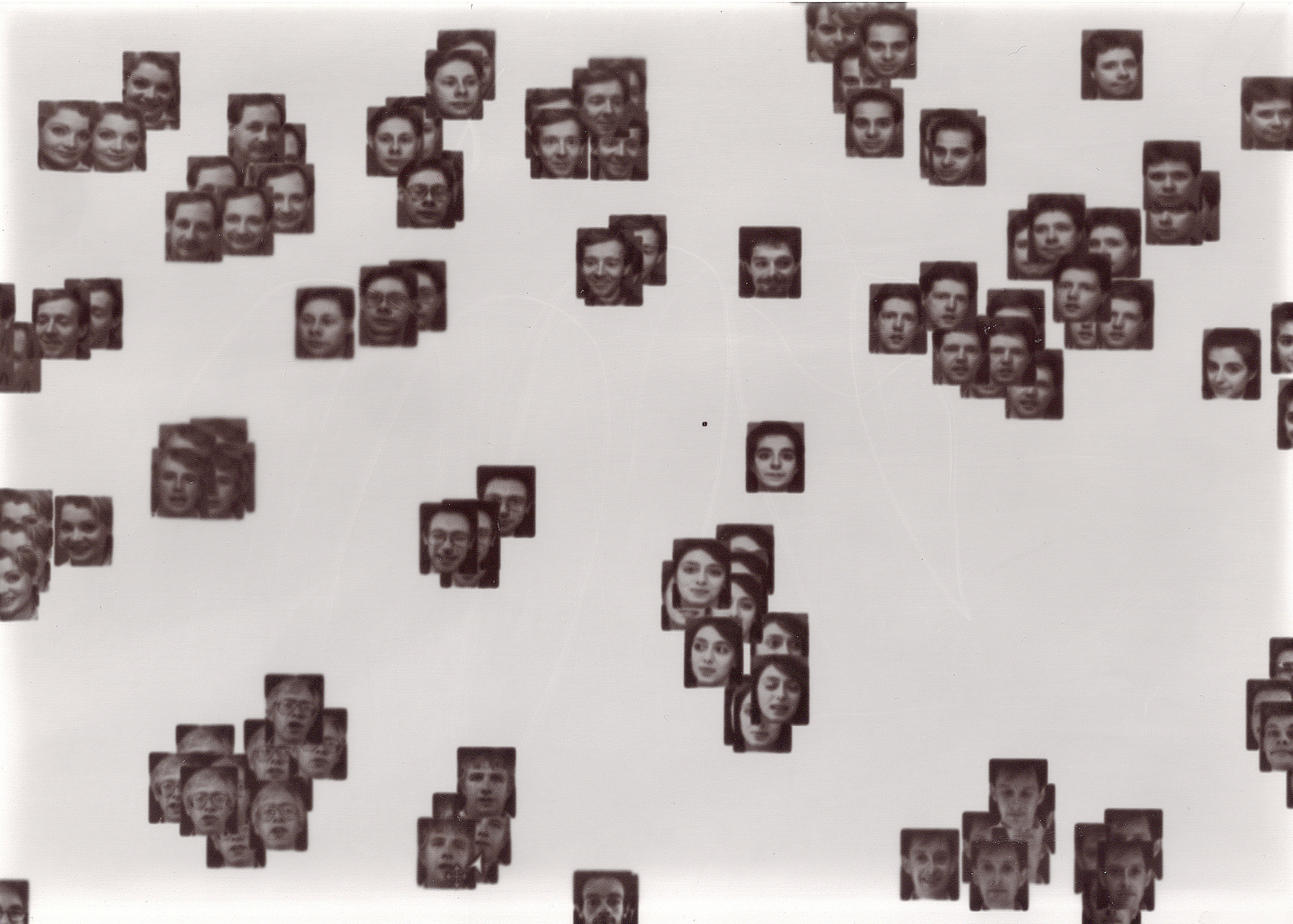
What are Recommender Systems?
Authors: Eduardo Magrani and Paula Guedes Fernandes da Silva In the current data-driven economy, Recommender Systems (RS), developed from…